This is a minimalistic Instagram scraper written in Python. It can fetch media, accounts, videos, comments etc. `Comment` and `Like` actions are also supported. It is not easy to get Applications approved for Instagram's API therefore I created this tool inspired by instagram-php-scraper. Python social-media pinterest web-scraper web-scraping request instagram-scraper twitter-scraper facebook-scraper scrapping-python selenium-python reddit-scraper quora-scraper tiktok-scraper medium-scraper pinterest-scrapper. The reason why Python is a preferred language to use for web scraping is that Scrapy and Beautiful Soup are two of the most widely employed frameworks based on Python. Beautiful Soup- well, it is a Python library that is designed for fast and highly efficient data extraction.
- Python Best Web Scraper
- Create Web Scraper Python Tutorial
- Create Web Scraper Python Github
- Web Scraper Chrome Extension
Internet extends fast and modern websites pretty often use dynamic content load mechanisms to provide the best user experience. Still, on the other hand, it becomes harder to extract data from such web pages, as it requires the execution of internal Javascript in the page context while scraping. Let's review several conventional techniques that allow data extraction from dynamic websites using Python.
What is a dynamic website?#

A dynamic website is a type of website that can update or load content after the initial HTML load. So the browser receives basic HTML with JS and then loads content using received Javascript code. Such an approach allows increasing page load speed and prevents reloading the same layout each time you'd like to open a new page.
Usually, dynamic websites use AJAX to load content dynamically, or even the whole site is based on a Single-Page Application (SPA) technology.
In contrast to dynamic websites, we can observe static websites containing all the requested content on the page load.
A great example of a static website is example.com
:
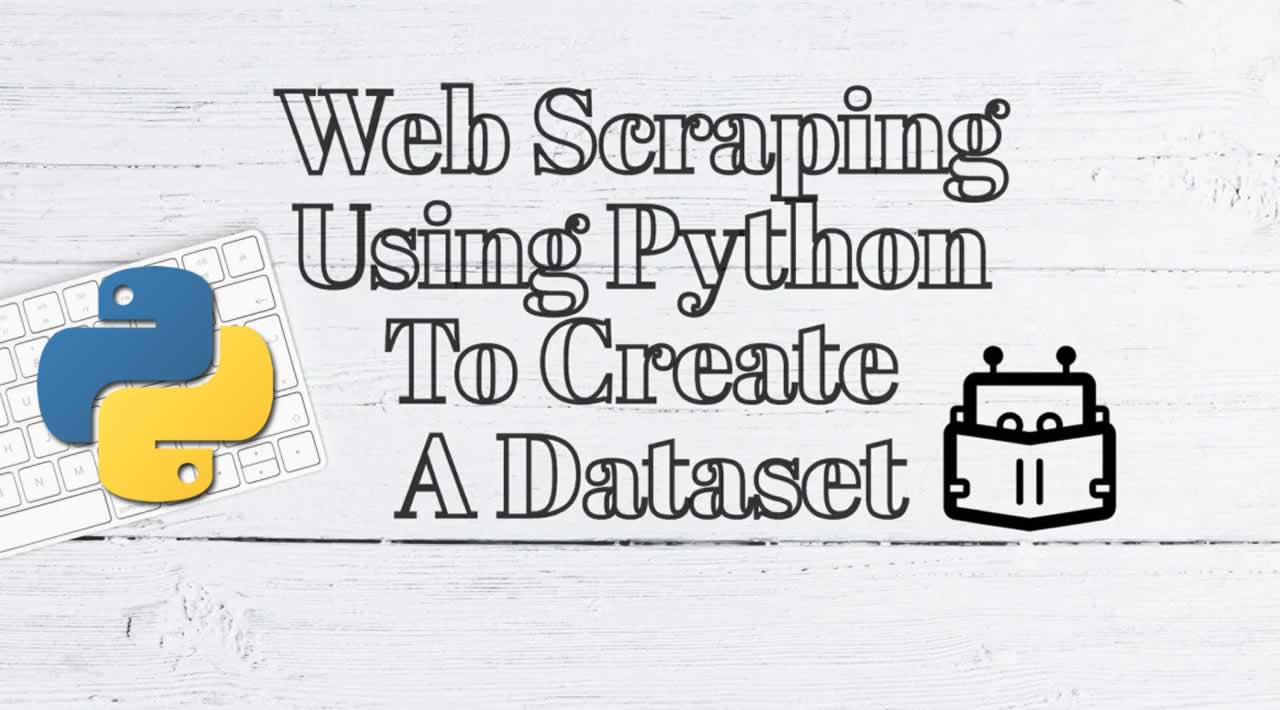
The whole content of this website is loaded as a plain HTML while the initial page load.
To demonstrate the basic idea of a dynamic website, we can create a web page that contains dynamically rendered text. It will not include any request to get information, just a render of a different HTML after the page load:
All we have here is an HTML file with a single <div>
in the body that contains text - Web Scraping is hard
, but after the page load, that text is replaced with the text generated by the Javascript:
To prove this, let's open this page in the browser and observe a dynamically replaced text:
Alright, so the browser displays a text, and HTML tags wrap this text.
Can't we use BeautifulSoup or LXML to parse it? Let's find out.
Extract data from a dynamic web page#
BeautifulSoup is one of the most popular Python libraries across the Internet for HTML parsing. Almost 80% of web scraping Python tutorials use this library to extract required content from the HTML.
Let's use BeautifulSoup for extracting the text inside <div>
from our sample above.
This code snippet uses os
library to open our test HTML file (test.html
) from the local directory and creates an instance of the BeautifulSoup library stored in soup
variable. Using the soup
we find the tag with id test
and extracts text from it.

In the screenshot from the first article part, we've seen that the content of the test page is I ❤️ ScrapingAnt
, but the code snippet output is the following:
And the result is different from our expectation (except you've already found out what is going on there). Everything is correct from the BeautifulSoup perspective - it parsed the data from the provided HTML file, but we want to get the same result as the browser renders. The reason is in the dynamic Javascript that not been executed during HTML parsing.
We need the HTML to be run in a browser to see the correct values and then be able to capture those values programmatically.
Below you can find four different ways to execute dynamic website's Javascript and provide valid data for an HTML parser: Selenium, Pyppeteer, Playwright, and Web Scraping API.
Selenuim: web scraping with a webdriver#
Selenium is one of the most popular web browser automation tools for Python. It allows communication with different web browsers by using a special connector - a webdriver.
To use Selenium with Chrome/Chromium, we'll need to download webdriver from the repository and place it into the project folder. Don't forget to install Selenium itself by executing:
Selenium instantiating and scraping flow is the following:
- define and setup Chrome path variable
- define and setup Chrome webdriver path variable
- define browser launch arguments (to use headless mode, proxy, etc.)
- instantiate a webdriver with defined above options
- load a webpage via instantiated webdriver
In the code perspective, it looks the following:

And finally, we'll receive the required result:
Selenium usage for dynamic website scraping with Python is not complicated and allows you to choose a specific browser with its version but consists of several moving components that should be maintained. The code itself contains some boilerplate parts like the setup of the browser, webdriver, etc.
I like to use Selenium for my web scraping project, but you can find easier ways to extract data from dynamic web pages below.
Pyppeteer: Python headless Chrome#
Pyppeteer is an unofficial Python port of Puppeteer JavaScript (headless) Chrome/Chromium browser automation library. It is capable of mainly doing the same as Puppeteer can, but using Python instead of NodeJS.
Puppeteer is a high-level API to control headless Chrome, so it allows you to automate actions you're doing manually with the browser: copy page's text, download images, save page as HTML, PDF, etc.
To install Pyppeteer you can execute the following command:
The usage of Pyppeteer for our needs is much simpler than Selenium:
I've tried to comment on every atomic part of the code for a better understanding. However, generally, we've just opened a browser page, loaded a local HTML file into it, and extracted the final rendered HTML for further BeautifulSoup processing.
As we can expect, the result is the following:
We did it again and not worried about finding, downloading, and connecting webdriver to a browser. Though, Pyppeteer looks abandoned and not properly maintained. This situation may change in the nearest future, but I'd suggest looking at the more powerful library.
Playwright: Chromium, Firefox and Webkit browser automation#
Playwright can be considered as an extended Puppeteer, as it allows using more browser types (Chromium, Firefox, and Webkit) to automate modern web app testing and scraping. You can use Playwright API in JavaScript & TypeScript, Python, C# and, Java. And it's excellent, as the original Playwright maintainers support Python.
The API is almost the same as for Pyppeteer, but have sync and async version both.
Installation is simple as always:
Let's rewrite the previous example using Playwright.
As a good tradition, we can observe our beloved output:
We've gone through several different data extraction methods with Python, but is there any more straightforward way to implement this job? How can we scale our solution and scrape data with several threads?
Meet the web scraping API!
Web Scraping API#
ScrapingAnt web scraping API provides an ability to scrape dynamic websites with only a single API call. It already handles headless Chrome and rotating proxies, so the response provided will already consist of Javascript rendered content. ScrapingAnt's proxy poll prevents blocking and provides a constant and high data extraction success rate.
Usage of web scraping API is the simplest option and requires only basic programming skills.
You do not need to maintain the browser, library, proxies, webdrivers, or every other aspect of web scraper and focus on the most exciting part of the work - data analysis.
As the web scraping API runs on the cloud servers, we have to serve our file somewhere to test it. I've created a repository with a single file: https://github.com/kami4ka/dynamic-website-example/blob/main/index.html
To check it out as HTML, we can use another great tool: HTMLPreview
The final test URL to scrape a dynamic web data has a following look: http://htmlpreview.github.io/?https://github.com/kami4ka/dynamic-website-example/blob/main/index.html
The scraping code itself is the simplest one across all four described libraries. We'll use ScrapingAntClient library to access the web scraping API.
Let's install in first:
And use the installed library:
Python Best Web Scraper
To get you API token, please, visit Login page to authorize in ScrapingAnt User panel. It's free.
And the result is still the required one.
All the headless browser magic happens in the cloud, so you need to make an API call to get the result.
Check out the documentation for more info about ScrapingAnt API.
Summary#
Today we've checked four free tools that allow scraping dynamic websites with Python. All these libraries use a headless browser (or API with a headless browser) under the hood to correctly render the internal Javascript inside an HTML page. Below you can find links to find out more information about those tools and choose the handiest one:
Happy web scraping, and don't forget to use proxies to avoid blocking 🚀
Web crawling is a powerful technique to collect data from the web by finding all the URLs for one or multiple domains. Python has several popular web crawling libraries and frameworks.
In this article, we will first introduce different crawling strategies and use cases. Then we will build a simple web crawler from scratch in Python using two libraries: requests and Beautiful Soup. Next, we will see why it’s better to use a web crawling framework like Scrapy. Finally, we will build an example crawler with Scrapy to collect film metadata from IMDb and see how Scrapy scales to websites with several million pages.
What is a web crawler?
Web crawling and web scraping are two different but related concepts. Web crawling is a component of web scraping, the crawler logic finds URLs to be processed by the scraper code.
A web crawler starts with a list of URLs to visit, called the seed. For each URL, the crawler finds links in the HTML, filters those links based on some criteria and adds the new links to a queue. All the HTML or some specific information is extracted to be processed by a different pipeline.
Web crawling strategies
In practice, web crawlers only visit a subset of pages depending on the crawler budget, which can be a maximum number of pages per domain, depth or execution time.
Most popular websites provide a robots.txt file to indicate which areas of the website are disallowed to crawl by each user agent. The opposite of the robots file is the sitemap.xml file, that lists the pages that can be crawled.
Popular web crawler use cases include:
- Search engines (Googlebot, Bingbot, Yandex Bot…) collect all the HTML for a significant part of the Web. This data is indexed to make it searchable.
- SEO analytics tools on top of collecting the HTML also collect metadata like the response time, response status to detect broken pages and the links between different domains to collect backlinks.
- Price monitoring tools crawl e-commerce websites to find product pages and extract metadata, notably the price. Product pages are then periodically revisited.
- Common Crawl maintains an open repository of web crawl data. For example, the archive from October 2020 contains 2.71 billion web pages.
Next, we will compare three different strategies for building a web crawler in Python. First, using only standard libraries, then third party libraries for making HTTP requests and parsing HTML and finally, a web crawling framework.
Building a simple web crawler in Python from scratch
To build a simple web crawler in Python we need at least one library to download the HTML from a URL and an HTML parsing library to extract links. Python provides standard libraries urllib for making HTTP requests and html.parser for parsing HTML. An example Python crawler built only with standard libraries can be found on Github.
The standard Python libraries for requests and HTML parsing are not very developer-friendly. Other popular libraries like requests, branded as HTTP for humans, and Beautiful Soup provide a better developer experience. You can install the two libraries locally.
A basic crawler can be built following the previous architecture diagram.
The code above defines a Crawler class with helper methods to download_url using the requests library, get_linked_urls using the Beautiful Soup library and add_url_to_visit to filter URLs. The URLs to visit and the visited URLs are stored in two separate lists. You can run the crawler on your terminal.
The crawler logs one line for each visited URL.
The code is very simple but there are many performance and usability issues to solve before successfully crawling a complete website.
- The crawler is slow and supports no parallelism. As can be seen from the timestamps, it takes about one second to crawl each URL. Each time the crawler makes a request it waits for the request to be resolved and no work is done in between.
- The download URL logic has no retry mechanism, the URL queue is not a real queue and not very efficient with a high number of URLs.
- The link extraction logic doesn’t support standardizing URLs by removing URL query string parameters, doesn’t handle URLs starting with #, doesn’t support filtering URLs by domain or filtering out requests to static files.
- The crawler doesn’t identify itself and ignores the robots.txt file.
Next, we will see how Scrapy provides all these functionalities and makes it easy to extend for your custom crawls.
Web crawling with Scrapy
Create Web Scraper Python Tutorial
Scrapy is the most popular web scraping and crawling Python framework with 40k stars on Github. One of the advantages of Scrapy is that requests are scheduled and handled asynchronously. This means that Scrapy can send another request before the previous one is completed or do some other work in between. Scrapy can handle many concurrent requests but can also be configured to respect the websites with custom settings, as we’ll see later.
Scrapy has a multi-component architecture. Normally, you will implement at least two different classes: Spider and Pipeline. Web scraping can be thought of as an ETL where you extract data from the web and load it to your own storage. Spiders extract the data and pipelines load it into the storage. Transformation can happen both in spiders and pipelines, but I recommend that you set a custom Scrapy pipeline to transform each item independently of each other. This way, failing to process an item has no effect on other items.
On top of all that, you can add spider and downloader middlewares in between components as it can be seen in the diagram below.
Scrapy Architecture Overview [source]
If you have used Scrapy before, you know that a web scraper is defined as a class that inherits from the base Spider class and implements a parse method to handle each response. If you are new to Scrapy, you can read this article for easy scraping with Scrapy.
Scrapy also provides several generic spider classes: CrawlSpider, XMLFeedSpider, CSVFeedSpider and SitemapSpider. The CrawlSpider class inherits from the base Spider class and provides an extra rules attribute to define how to crawl a website. Each rule uses a LinkExtractor to specify which links are extracted from each page. Next, we will see how to use each one of them by building a crawler for IMDb, the Internet Movie Database.
Building an example Scrapy crawler for IMDb
Before trying to crawl IMDb, I checked IMDb robots.txt file to see which URL paths are allowed. The robots file only disallows 26 paths for all user-agents. Scrapy reads the robots.txt file beforehand and respects it when the ROBOTSTXT_OBEY setting is set to true. This is the case for all projects generated with the Scrapy command startproject.
This command creates a new project with the default Scrapy project folder structure.
Then you can create a spider in scrapy_crawler/spiders/imdb.py with a rule to extract all links.
You can launch the crawler in the terminal.
You will get lots of logs, including one log for each request. Exploring the logs I noticed that even if we set allowed_domains to only crawl web pages under https://www.imdb.com, there were requests to external domains, such as amazon.com.
IMDb redirects from URLs paths under whitelist-offsite and whitelist to external domains. There is an open Scrapy Github issue that shows that external URLs don’t get filtered out when the OffsiteMiddleware is applied before the RedirectMiddleware. To fix this issue, we can configure the link extractor to deny URLs starting with two regular expressions.
Rule and LinkExtractor classes support several arguments to filter out URLs. For example, you can ignore specific URL extensions and reduce the number of duplicate URLs by sorting query strings. If you don’t find a specific argument for your use case you can pass a custom function to process_links in LinkExtractor or process_values in Rule.
For example, IMDb has two different URLs with the same content.
To limit the number of crawled URLs, we can remove all query strings from URLs with the url_query_cleaner function from the w3lib library and use it in process_links.
Now that we have limited the number of requests to process, we can add a parse_item method to extract data from each page and pass it to a pipeline to store it. For example, we can either extract the whole response.text to process it in a different pipeline or select the HTML metadata. To select the HTML metadata in the header tag we can code our own XPATHs but I find it better to use a library, extruct, that extracts all metadata from an HTML page. You can install it with pip install extract.
I set the follow attribute to True so that Scrapy still follows all links from each response even if we provided a custom parse method. I also configured extruct to extract only Open Graph metadata and JSON-LD, a popular method for encoding linked data using JSON in the Web, used by IMDb. You can run the crawler and store items in JSON lines format to a file.
The output file imdb.jl contains one line for each crawled item. For example, the extracted Open Graph metadata for a movie taken from the <meta> tags in the HTML looks like this.
Create Web Scraper Python Github
The JSON-LD for a single item is too long to be included in the article, here is a sample of what Scrapy extracts from the <script type='application/ld+json'> tag.
Exploring the logs, I noticed another common issue with crawlers. By sequentially clicking on filters, the crawler generates URLs with the same content, only that the filters were applied in a different order.
Long filter and search URLs is a difficult problem that can be partially solved by limiting the length of URLs with a Scrapy setting, URLLENGTH_LIMIT.
Web Scraper Chrome Extension
I used IMDb as an example to show the basics of building a web crawler in Python. I didn’t let the crawler run for long as I didn’t have a specific use case for the data. In case you need specific data from IMDb, you can check the IMDb Datasets project that provides a daily export of IMDb data and IMDbPY, a Python package for retrieving and managing the data.
Web crawling at scale
If you attempt to crawl a big website like IMDb, with over 45M pages based on Google, it’s important to crawl responsibly by configuring the following settings. You can identify your crawler and provide contact details in the BOT_NAME setting. To limit the pressure you put on the website servers you can increase the DOWNLOAD_DELAY, limit the CONCURRENT_REQUESTS_PER_DOMAIN or set AUTOTHROTTLE_ENABLED that will adapt those settings dynamically based on the response times from the server.
Notice that Scrapy crawls are optimized for a single domain by default. If you are crawling multiple domains check these settings to optimize for broad crawls, including changing the default crawl order from depth-first to breath-first. To limit your crawl budget, you can limit the number of requests with the CLOSESPIDER_PAGECOUNT setting of the close spider extension.
With the default settings, Scrapy crawls about 600 pages per minute for a website like IMDb. To crawl 45M pages it will take more than 50 days for a single robot. If you need to crawl multiple websites it can be better to launch separate crawlers for each big website or group of websites. If you are interested in distributed web crawls, you can read how a developer crawled 250M pages with Python in 40 hours using 20 Amazon EC2 machine instances.
In some cases, you may run into websites that require you to execute JavaScript code to render all the HTML. Fail to do so, and you may not collect all links on the website. Because nowadays it’s very common for websites to render content dynamically in the browser I wrote a Scrapy middleware for rendering JavaScript pages using ScrapingBee’s API.
Conclusion
We compared the code of a Python crawler using third-party libraries for downloading URLs and parsing HTML with a crawler built using a popular web crawling framework. Scrapy is a very performant web crawling framework and it’s easy to extend with your custom code. But you need to know all the places where you can hook your own code and the settings for each component.
Configuring Scrapy properly becomes even more important when crawling websites with millions of pages. If you want to learn more about web crawling I suggest that you pick a popular website and try to crawl it. You will definitely run into new issues, which makes the topic fascinating!
Sources
